AIDA is dead! Well, almost. The strictly linear concept of Elmo Lewis’s AIDA step model has become less important as digitalization in marketing progresses. Nevertheless, it still makes sense to analyze AdWords Search Funnel along the four steps: attention, interest, desire and action. In this blog post, we show how this analysis can increase AdWords performance.
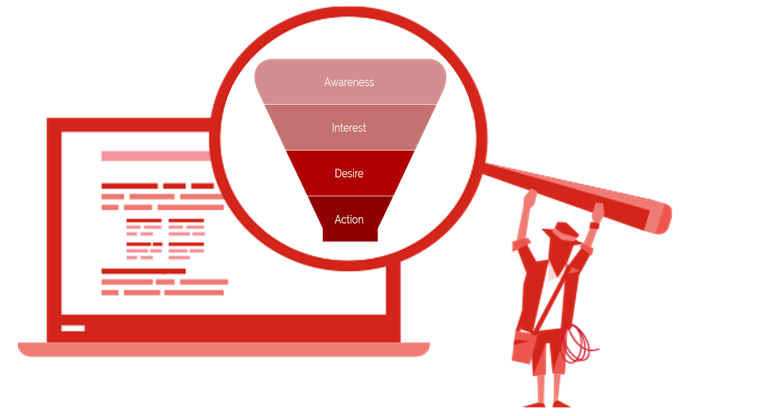
Was bringt Semantikanalyse mit AIDA?
For some time now, digital marketing specialists have been calling for attribution models for performance analysis of their campaigns, in order evaluate individual touchpoints along the customer journey and attribute conversions for a specified model to channels. As a rule, they focus on cross-channel analysis.
However, an isolated assessment of AdWords Search Funnels using the AIDA step model is also worthwhile, especially when it comes to examining the value and function of keywords in Search Funnels and optimizing overall performance – because first and last click attribution models provide only an incomplete picture of performance.
This assumption is based on extensive data analysis. We allocated the keywords and search terms of a major Swiss e-commerce platform’s AdWords account across the four AIDA steps. We then tracked the performance of the semantically similar keywords in each defined step over the course of a year.
AIDA keyword steps – an example
The search campaign for Stieg Larsson’s book, The Girl in the Spider’s Web, will help us shine light on our experiment. The overall objective of this campaign is to encourage customers to buy the new book. To analyze the performance and function of each keyword and search term in this search campaign, they were allocated across the AIDA steps.
The first step, attention, includes all keywords and search terms that focuses the attention of the user on a topic that may be of interest. In this specific example, the user is looking for new book releases because they like to read. Sample keywords: new book releases, new books, book publications, current bestseller
The second step, interest, includes all keywords and search terms that a user uses to follow up their aroused interest. If the user likes to read novels by Stieg Larsson, for example, they may look for reviews of the author’s latest book. Sample keywords: The Girl in the Spider’s Web review, book review Stieg Larsson, book review The Girl in the Spider’s Web, critique The Girl in the Spider’s Web
The third step, desire, includes all keywords and search terms through which the user expresses a specific desire to buy the product. For instance, they search for retailers, comparison portals or prices. In our example, the user is interested in purchasing Stieg Larsson’s The Girl in the Spider’s Web. Sample keywords: price The Girl in the Spider’s Web, online book store The Girl in the Spider’s Web, The Girl in the Spider’s Web Amazon
The final step, action, includes all keywords and search terms through which the user expresses an intention to buy the product. The user has decided to purchase The Girl in the Spider’s Web after an in-depth consideration of the purchasing options. Sample keywords: buy The Girl in the Spider’s Web online, The Girl in the Spider’s web buy postage free, or The Girl in the Spider’s Web
Performance analysis – the facts
After the keywords and search terms have been allocated to the various steps of the model, the next stage is the performance analysis. A clear picture emerges from analysis of our e-commerce customer’s data sets: in terms of cost-per-conversion and conversion rates, performance continues to improve for both keywords and search terms as the user progresses through the steps.
From step 1 (attention) to step 4 (action), performance improved 10% in terms of conversion rate and 20% with respect to cost-per-conversion. This is to be expected as the intention and thus the likelihood of a conversion rises along the Search Funnel. It follows that a shift of click volume towards steps 3 and 4 may lead to a boost in performance for AdWords accounts with budget constraints.
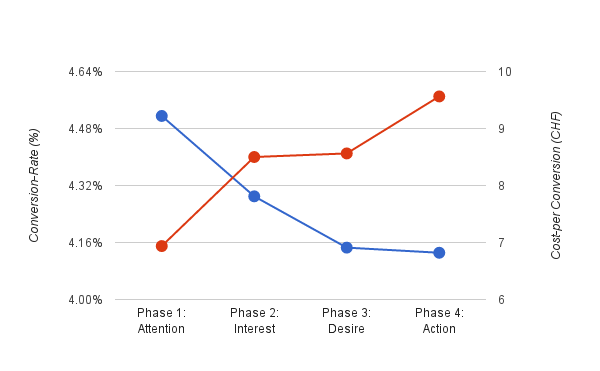
Graph Semantikanalyse
image: screenshot analysis, red = Conversion Rate, blue = Cost per Conversion
This kind of analysis is particularly useful for search marketers in establishing the relationship between first clicks and last clicks. In our analysis, the specific ratio of steps 1 and 2 to steps 3 and 4 was 21%. In other words, keywords allocated to steps 1 and 2 generated about one fifth of click volume. Search marketers must ask themselves how they want to apportion their budget between the first and last clicks, although obviously this will vary widely, depending on the AdWords account’s performance and goals.
A predefined keyword grid can help identify and exclude underperforming search terms and keywords in each step of the AIDA model relatively easily. For AdWords accounts with large keyword volumes, this can lead to a rapid increase in performance.
Our conclusion
Finally, an isolated analysis of keywords using the AIDA model proposed here represents only a part of reality; in particular, cross-channel classification and evaluation of AdWords traffic is missing. Therefore, before excluding or pausing a keyword, the overall context should be considered and analyzed in terms of its assistive value.
Furthermore, the strict separation of the search phases in the linear four-step model must be approached with caution: decision-making processes are increasingly non-linear in nature, as users jump back and forth between the four steps and are addressed several times on different channels. Nevertheless, the AIDA model offers a good set of guidelines for analysis of AdWords accounts, and provides a solid starting point for more in-depth keyword analysis. For AdWords accounts with budget constraints and high performance goals in particular, application of the AIDA model may lead to a single-digit performance increase.